Introduction
Many factors can contribute to the development of COPD. While cigarette smoking is the number one risk factor for COPD in the United States, recent research has indicated that genes may also play a significant role and could be responsible for why some people who smoke will develop COPD while others will not. In addition, these genetic risk factors (or others) could influence why some nonsmokers also can develop COPD. The marked variability in lung function and risk of COPD in people with similar cigarette smoking histories, together with studies of familial aggregation, support an important role for genetics in COPD. Multiple review articles have summarized the state of the art for COPD genetics research, in general,1, 2 and from COPDGene, in particular.3 Below, we provide a general overview of this research area.
Performing a Genetic Study
Doing scientific research involves the use of multiple tools and techniques in order to discover new information. Researchers employ different methods to conduct a study depending on what is being studied and what type of information is being sought. At the COPDGene Study, we are looking for genes that might be involved in the development and disease progression of COPD.
The COPDGene Study is a type of epidemiological study, called a genetic epidemiology study. Epidemiological research looks at a large population of individuals and tries to understand a disease process within that population. A genetic epidemiological study looks for genes that are suspected to be involved in a disease process for a population. The general goal of a genetic epidemiological study is to identify genes that affect the health and wellness of a population.
Conducting a genetic epidemiological study like COPDGene requires following a well-defined research protocol that will help reduce the collection of irrelevant or inaccurate data. The general method used in COPDGene is outlined below.
1. Identify a study population
When scientists attempt to look for genes that are associated with a particular trait, they first have to choose a population that expresses that trait. In order to do this, a population must be chosen that uniquely fits a physical or behavioral model that will help separate the population into groups that share more characteristics. For instance, men and women are often separated into two populations or groups in a study because of differences in physiological traits. People from different ancestry backgrounds are also often studied as unique groups as some genes may be exclusively expressed for these groups. For instance, people of Northern European descent tend to carry the gene for alpha-1 antitrypsin deficiency more commonly than people from other ancestry groups.
Most genetic epidemiology studies need at least two distinct populations: a control group and a case group. A control group is chosen to serve as a ‘normal’ or unchanged population, and the case group is the population that shares a particular trait or feature of interest that is being studied. For instance, if researchers wanted to understand how smoking affects the lungs, they would study the lungs of a group of smokers (cases) and the lungs of non-smokers (controls) looking for differences between the two groups. In a genetic study, researchers look for people who share certain disease features and try to find variants in genes that are common to that group but that may not be found as often in the control population. The traits that are used to collect a study group have been carefully selected and will help narrow the genetic diversity of the population, thus making finding genetic similarities more likely. The COPDGene Study looks for people with specific smoking histories, age, ancestry, gender, lung health, and several other factors. By gathering a specific population, genes that may be associated with a disease can be more easily found. In addition to case-control studies, quantitative disease-related characteristics can be studied in large populations, without separating subjects into case and control groups. Family-based studies can also be used with either categorical (e.g., case vs. control) or quantitative (e.g., lung function level) outcomes. Both case-control and quantitative population-based quantitative genetic analyses have been performed in COPDGene.
2. Measure key characteristics of the members of the study populations
The next step after finding a group of people that fit specified criteria is to measure their disease-related characteristics. Taking measurements helps to further define a population and helps to outline the specific traits and features that they share. In COPDGene, measurements that relate to COPD, lung health, and general physical health are taken. These measurements can then be used to assess the severity of disease and place study participants into smaller groups based on their lung health. At a COPDGene Study visit, a chest CT scan is performed to get a visual assessment of lung disease, a walk test is performed, spirometry data are collected, medical history is recorded, and blood is taken. The blood samples are used to extract DNA and study genes.
Each of the measurements taken will help scientists study correlations between factors and draw conclusions about disease states. For instance, by measuring how much people smoke and the health of their lungs, it has been concluded that people who smoke cigarettes have a higher risk of developing lung disease than non-smokers.
3. Look for genetic similarities
The next step in a genetic epidemiology study is to look for genetic variants that differ between the case and control populations. This requires the aid of biostatisticians and molecular geneticists to find genetic associations. Most genes associated with disease are not easy to find because each gene may only contribute a small portion of the total genetic component of a disease. Unlike more clear-cut conditions with one primary gene responsible for a trait (like those that are responsible for cystic fibrosis or blood type), many diseases are caused by multiple genes of small effect that function together to create a complex array of physiological processes leading to disease. Finding all the parts and assessing their importance with respect to the disease is difficult and often does not produce definitive results.
Further complicating matters is the problem of genes interacting with the environment, which may change which genes are expressed. ‘The environment’ refers to factors that a person is exposed to outside of their physical body that can change the way the body functions. For instance, if genetic factors are found for COPD, simply having the genes may not be enough to develop the disease. Smoking cigarettes, however, could interact with the COPD genes potentially causing COPD.
The main way in which the COPDGene Study looks for genes within the study population is through the use of a Genome Wide Association Study, or GWAS for short. A genome-wide association study is a scientific approach that involves scanning hundreds of thousands of genetic markers across the complete sets of DNA, or genomes, of many people to find genetic variations associated with a particular disease. Either a standard panel of genetic markers or sequencing of the entire genome can be used to determine an individual’s genetic variants. GWAS studies have been frequently used as a tool to find genes involved in complex diseases such as COPD, heart disease, and cancer.
The overall approach for GWAS is shown in the figure below, which was originally published in the Journal of the COPD Foundation.4 After enrolling and measuring characteristics of the study population, standardized genome-wide single nucleotide polymorphism (SNP) genotyping of panels including hundreds of thousands of genetic variants is performed. Quality control is performed at the level of the study subject (excluding subjects with high rates of missing genotypes, suggesting low quality DNA samples, or gender inconsistencies, suggesting possible sample mix-ups) and the level of the genetic marker (excluding markers with high rates of missing data, deviations from expected genotype distributions in control subjects, etc.). Genetic association analysis is performed with regression analysis (e.g., logistic regression for categorical phenotypes and multiple regression for quantitative phenotypes). The genotyped SNPs can be utilized to impute likely genotypes at other SNPs for which they are correlated (termed linkage disequilibrium) by using statistical imputation approaches with standard reference panels. Due to the large number of genetic variants tested, stringent adjustment for multiple statistical testing is required, with p-values < 5 x 10-8 typically utilized to demonstrate genome-wide significance. Meta-analysis of multiple study populations is often required to achieve statistical significance, and replication of association results substantially increases confidence in the validity of the associations.
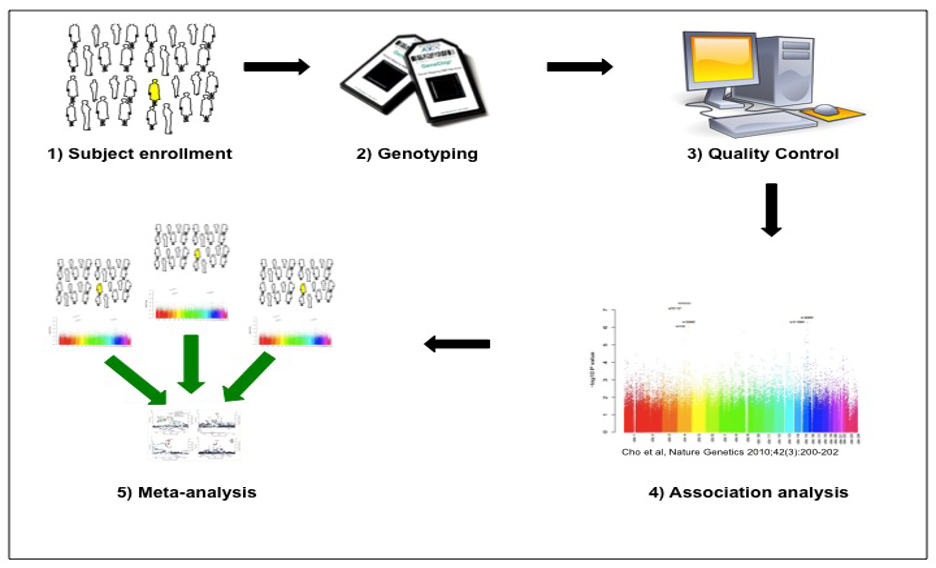
4. Make conclusions
After all the information is collected, scientists begin to make conclusions about what was found. In a genetics study, this often involves making conclusions about trends seen in the data. Discovering a trend or an association of a gene with a particular disease or even one aspect of a disease does not mean that every individual that has that gene will develop the disease. This is where the idea of ‘risk factors’ come into play. Say for instance, a random group of 100 people is studied for genetic similarities and it is found that 50 of the people studied have a particular set of genes in common. Of those 50 people, 40 also have COPD. The conclusion one might make is that these particular genes may be associated with COPD, though it could not be said that they definitively cause COPD as 10 people in the group who have the genes do not have COPD. Drawing conclusions about genetic similarities works in the same way; genes are often associated with a disease but do not always absolutely cause it.
So what accounts for the differences then? While it is not completely understood why some people with a disease-associated gene will develop the disease when others may not, it is widely accepted that environmental factors play an important role. The process of genetic and environmental risk factors working together to cause an outcome is commonly referred to as the gene-environment interaction. In short, genes may set the stage for a disease to occur, but only through environmental exposures acting in concert with the genes will a disease actually develop.
Current Status of COPD Genetics Research
The marked variability in lung function and risk for COPD in people with similar cigarette smoking histories, together with studies of familial aggregation, support an important role for genetic risk factors in COPD. A small but important fraction of COPD cases harbor a major genetic determinant, α1-antitrypsin deficiency (AATD). This condition is most common in populations of Northern European ancestry, although affected individuals in other populations can be found. Despite significant advances in diagnosis and treatment, AATD remains highly under-diagnosed. Manifestations other than classic lower-lobe predominant emphysema can include bronchiectasis, liver disease, panniculitis, and vasculitis. Intravenous augmentation with AAT protein is a commonly used treatment for severe AATD. Individuals who inherit two abnormal AAT genes (one from each parent) are at substantially increased risk for COPD, but individuals who inherit one abnormal AAT gene are also at some increased risk for COPD if they smoke.5
The discovery of alpha-1 antitrypsin (AAT) deficiency was a major factor in developing the Protease-Antiprotease Hypothesis for COPD, a prevailing model of disease pathogenesis for over 40 years. Hence, it was natural to hope that the identification of other COPD susceptibility genes would lead to similar novel insights into the causes of COPD. However, the results of many candidate gene association studies were been largely inconsistent6. These inconsistencies likely relate to a variety of methodological issues, including small sample sizes, failure to adjust for multiple statistical testing, variability in disease characterization, and inadequate adjustment for population stratification. However, the greatest problem in these studies likely was improper candidate gene selection, reflecting our limited understanding of COPD pathogenesis. By contrast, the application of genome-wide association studies (GWAS), which provide an unbiased and comprehensive search throughout the genome for common susceptibility loci, has changed the landscape of COPD genetics. Based on GWAS, 82 novel genetic loci have been unequivocally associated with COPD susceptibility as shown in the figure below. The horizontal axis shows the 22 autosomal chromosomes, and the vertical axis shows the strength of association to COPD, which is expressed as –log10(p-value). This work was based on a collaborative study between the International COPD Genetics Consortium (including COPDGene) and the UK Biobank; 35,735 COPD cases and 222,076 controls from 24 studies were included.7 The red dotted line corresponds to the level of statistical significance adjusting for testing so many genetic variants (p=5 x 10-8), and the labels provide gene names closest to the new associations described in that article. For these 35 new associations, replication in the SpiroMeta study is indicated.
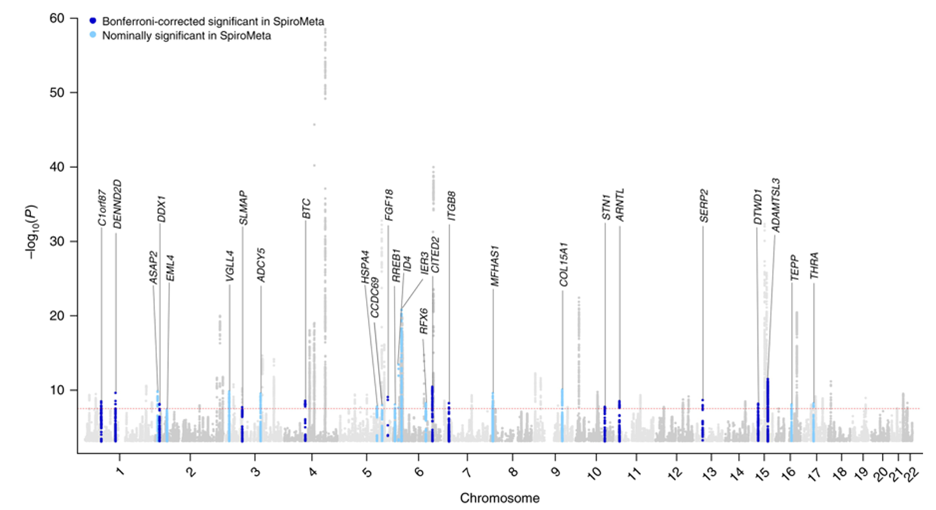
Although these genetic associations identify regions of the genome related to COPD risk, additional work is required to identify the functional genetic variants and the genes that they influence within these genomic regions. Most complex disease GWAS determinants influence gene regulation rather than altering the protein coding sequence of a gene. Approaches such as chromosome conformation capture8 and massively parallel reporter assays9 have been used to implicate likely functional variants and key genes in COPD GWAS regions. Genetically targeted mouse models (e.g., knock-out mice) that have alterations in emphysema susceptibility after chronic smoke exposure also provide support that a gene located within a COPD GWAS region is likely a key gene in that region influencing COPD susceptibility. Based on various types of functional studies, reasonable evidence has emerged that HHIP, FAM13A, AGER, FBNLN5, SFTPD, TET2, IREB2, MFAP2, DSP, FBXO38, NPNT, TGFB2, and MMP12 are likely COPD susceptibility genes within COPD GWAS regions.
In addition to genetic studies of the presence or absence of COPD, multiple disease-related characteristics have also been studied for genetic associations. For example, more than 1000 genomic regions have been associated with lung function levels.10
Although individual COPD GWAS variants explain a small portion of COPD genetic risk, combining multiple genetic variants into polygenic risk scores (PRSs) can substantially improve prediction. Moll and colleagues created a COPD PRS by combining association evidence to lung function (FEV1 and FEV1/FVC) across the genome in the UK Biobank and SpiroMeta studies. The PRS, which included hundreds of thousands of genetic variants, was then tested in nine other cohorts, including COPDGene. Each standard deviation increase in this risk score was associated with COPD with an odds ratio of 1.8 in Europeans and 1.4 in non-Europeans. Comparing across population deciles shown in the figure below, being in the tenth decile of polygenic risk was associated with an 8-fold odds of COPD compared to being in the first decile of predicted genetic risk in European ancestry individuals.

Thus, substantial progress has been made in understanding the genetic risk of COPD, but additional research is required to find more of the key genes in the identified genomic regions and to find new determinants of COPD progression. GWAS studies focus on common genetic variants that contribute to disease risk. Rare variants are being studied through DNA sequencing in the NHLBI Trans-Omics for Precision Medicine Program; rare variants likely also contribute to COPD susceptibility and are becoming an increasingly important focus of COPDGene.
REFERENCES
1. Silverman EK. Genetics of COPD. Annu Rev Physiol. 2020;82:413-31. Epub 2019/11/16. doi: 10.1146/annurev-physiol-021317-121224. PubMed PMID: 31730394.
2. Cho MH, Hobbs BD, Silverman EK. Genetics of chronic obstructive pulmonary disease: understanding the pathobiology and heterogeneity of a complex disorder. Lancet Respir Med. 2022;10(5):485-96. Epub 2022/04/16. doi: 10.1016/S2213-2600(21)00510-5. PubMed PMID: 35427534.
3. Ragland MF, Benway CJ, Lutz SM, Bowler RP, Hecker J, Hokanson JE, Crapo JD, Castaldi PJ, DeMeo DL, Hersh CP, Hobbs BD, Lange C, Beaty TH, Cho MH, Silverman EK. Genetic Advances in Chronic Obstructive Pulmonary Disease: Insights from COPDGene. Am J Respir Crit Care Med. 2019;200:677-90. Epub 2019/03/26. doi: 10.1164/rccm.201808-1455SO. PubMed PMID: 30908940.
4. Hardin M, Silverman EK. Chronic Obstructive Pulmonary Disease genetics: A review of the past and a look into the future. Journal of the COPD Foundation. 2014;1(1):33-46.
5. Foreman MG, Wilson C, DeMeo DL, Hersh CP, Beaty TH, Cho MH, Ziniti J, Curran-Everett D, Criner G, Hokanson JE, Brantly M, Rouhani FN, Sandhaus RA, Crapo JD, Silverman EK, Genetic Epidemiology of CI. Alpha-1 Antitrypsin PiMZ Genotype Is Associated with Chronic Obstructive Pulmonary Disease in Two Racial Groups. Ann Am Thorac Soc. 2017;14(8):1280-7. doi: 10.1513/AnnalsATS.201611-838OC. PubMed PMID: 28380308; PMCID: PMC5566271.
6. Castaldi PJ, Cho MH, Cohn M, Langerman F, Moran S, Tarragona N, Moukhachen H, Venugopal R, Hasimja D, Kao E, Wallace B, Hersh CP, Bagade S, Bertram L, Silverman EK, Trikalinos TA. The COPD genetic association compendium: a comprehensive online database of COPD genetic associations. Hum Mol Genet. 2010;19(3):526-34. Epub 2009/11/26. doi: 10.1093/hmg/ddp519. PubMed PMID: 19933216.
7. Sakornsakolpat P, Prokopenko D, Lamontagne M, Reeve NF, Guyatt AL, Jackson VE, Shrine N, Qiao D, Bartz TM, Kim DK, Lee MK, Latourelle JC, Li X, Morrow JD, Obeidat M, Wyss AB, Bakke P, Barr RG, Beaty TH, Belinsky SA, Brusselle GG, Crapo JD, de Jong K, DeMeo DL, Fingerlin TE, Gharib SA, Gulsvik A, Hall IP, Hokanson JE, Kim WJ, Lomas DA, London SJ, Meyers DA, O’Connor GT, Rennard SI, Schwartz DA, Sliwinski P, Sparrow D, Strachan DP, Tal-Singer R, Tesfaigzi Y, Vestbo J, Vonk JM, Yim JJ, Zhou X, Bosse Y, Manichaikul A, Lahousse L, Silverman EK, Boezen HM, Wain LV, Tobin MD, Hobbs BD, Cho MH, SpiroMeta C, International CGC. Genetic landscape of chronic obstructive pulmonary disease identifies heterogeneous cell-type and phenotype associations. Nat Genet. 2019;51(3):494-505. Epub 2019/02/26. doi: 10.1038/s41588-018-0342-2. PubMed PMID: 30804561; PMCID: PMC6546635.
8. Zhou X, Baron RM, Hardin M, Cho MH, Zielinski J, Hawrylkiewicz I, Sliwinski P, Hersh CP, Mancini JD, Lu K, Thibault D, Donahue AL, Klanderman BJ, Rosner B, Raby BA, Lu Q, Geldart AM, Layne MD, Perrella MA, Weiss ST, Choi AM, Silverman EK. Identification of a chronic obstructive pulmonary disease genetic determinant that regulates HHIP. Hum Mol Genet. 2012;21(6):1325-35. Epub 2011/12/06. doi: 10.1093/hmg/ddr569. PubMed PMID: 22140090; PMCID: 3284120.
9. Castaldi PJ, Guo F, Qiao D, Du F, Naing ZZC, Li Y, Pham B, Mikkelsen TS, Cho MH, Silverman EK, Zhou X. Identification of Functional Variants in the FAM13A Chronic Obstructive Pulmonary Disease Genome-Wide Association Study Locus by Massively Parallel Reporter Assays. Am J Respir Crit Care Med. 2019;199(1):52-61. Epub 2018/08/07. doi: 10.1164/rccm.201802-0337OC. PubMed PMID: 30079747.
10. Shrine N, Izquierdo AG, Chen J, Packer R, Hall RJ, Guyatt AL, Batini C, Thompson RJ, Pavuluri C, Malik V, Hobbs BD, Moll M, Kim W, Tal-Singer R, Bakke P, Fawcett KA, John C, Coley K, Piga NN, Pozarickij A, Lin K, Millwood IY, Chen Z, Li L, China Kadoorie Biobank Collaborative G, Wijnant SRA, Lahousse L, Brusselle G, Uitterlinden AG, Manichaikul A, Oelsner EC, Rich SS, Barr RG, Kerr SM, Vitart V, Brown MR, Wielscher M, Imboden M, Jeong A, Bartz TM, Gharib SA, Flexeder C, Karrasch S, Gieger C, Peters A, Stubbe B, Hu X, Ortega VE, Meyers DA, Bleecker ER, Gabriel SB, Gupta N, Smith AV, Luan J, Zhao JH, Hansen AF, Langhammer A, Willer C, Bhatta L, Porteous D, Smith BH, Campbell A, Sofer T, Lee J, Daviglus ML, Yu B, Lim E, Xu H, O’Connor GT, Thareja G, Albagha OME, Qatar Genome Program Research C, Suhre K, Granell R, Faquih TO, Hiemstra PS, Slats AM, Mullin BH, Hui J, James A, Beilby J, Patasova K, Hysi P, Koskela JT, Wyss AB, Jin J, Sikdar S, Lee M, May-Wilson S, Pirastu N, Kentistou KA, Joshi PK, Timmers P, Williams AT, Free RC, Wang X, Morrison JL, Gilliland FD, Chen Z, Wang CA, Foong RE, Harris SE, Taylor A, Redmond P, Cook JP, Mahajan A, Lind L, Palviainen T, Lehtimaki T, Raitakari OT, Kaprio J, Rantanen T, Pietilainen KH, Cox SR, Pennell CE, Hall GL, Gauderman WJ, Brightling C, Wilson JF, Vasankari T, Laitinen T, Salomaa V, Mook-Kanamori DO, Timpson NJ, Zeggini E, Dupuis J, Hayward C, Brumpton B, Langenberg C, Weiss S, Homuth G, Schmidt CO, Probst-Hensch N, Jarvelin MR, Morrison AC, Polasek O, Rudan I, Lee JH, Sayers I, Rawlins EL, Dudbridge F, Silverman EK, Strachan DP, Walters RG, Morris AP, London SJ, Cho MH, Wain LV, Hall IP, Tobin MD. Multi-ancestry genome-wide association analyses improve resolution of genes and pathways influencing lung function and chronic obstructive pulmonary disease risk. Nat Genet. 2023;55(3):410-22. Epub 2023/03/15. doi: 10.1038/s41588-023-01314-0. PubMed PMID: 36914875; PMCID: PMC10011137.