Proteomics
What is proteomics: Proteomics research is like a detective mission for our body’s tiny helpers, proteins. Scientists use powerful tools to examine and understand all the proteins in a given system, like our lungs, to uncover clues about various diseases. Imagine proteins as the workers in a bustling factory, each with a unique job. Proteomics helps scientists identify these workers and understand how they change when something goes wrong, like in diseases such as cancer or diabetes. By decoding the protein puzzle, researchers aim to find new ways to detect diseases early, understand how they progress, and even develop targeted treatments that can specifically address the issues in our body’s intricate machinery, offering hope for improved health and well-being.
COPDGene is helping us discover important COPD risk proteins through proteomics: COPDGene researchers are actively exploring various protein biomarkers associated with Chronic Obstructive Pulmonary Disease (COPD). Here are some proteins that are biomarkers for COPD:
- sRAGE: People with lower levels of sRAGE have more emphysema and more progression of COPD.
- C-reactive protein (CRP): Elevated levels of CRP have been associated with inflammation in COPD, and it is considered a marker of systemic inflammation.
- Interleukins (ILs): Various interleukins, such as IL-6 and IL-8, play roles in inflammation and have been implicated in COPD. Elevated levels of these interleukins have been observed in individuals with COPD.
- Tumor Necrosis Factor-alpha (TNF-α): TNF-α is a pro-inflammatory cytokine that has been linked to COPD. It contributes to the inflammatory processes in the airways seen in COPD patients.
- Matrix Metalloproteinases (MMPs): MMPs are enzymes involved in tissue remodeling, and their dysregulation has been associated with COPD. Increased levels of certain MMPs have been found in COPD patients.
- Surfactant Proteins (SP-A and SP-D): Alterations in surfactant proteins have been linked to COPD, reflecting changes in lung structure and function.
- Club Cell Secretory Protein (CC16): CC16 is a protein produced by bronchial epithelial cells, and its levels in serum and sputum have been investigated as potential biomarkers for COPD.
- Alpha-1 Antitrypsin (AAT): AAT deficiency is a genetic risk factor for COPD, and monitoring AAT levels can be important in managing COPD in individuals with this deficiency.
- Fibrinogen: Elevated levels of fibrinogen, a protein involved in blood clotting, have been associated with an increased risk of exacerbations in COPD.
Finding protein biomarkers for COPD: It’s crucial to note that the field of biomarker research in COPD is extensive, and identifying reliable biomarkers for diagnosis, prognosis, and treatment response remains an active area of investigation. Researchers are continually working to uncover new markers and validate existing ones for clinical use. Always refer to the latest scientific literature for the most recent information on COPD biomarkers.
Combining multiple proteins to develop COPD protein risk scores: Proteomic risk scores represent a cutting-edge approach in biomedical research, harnessing advanced proteomics technologies to evaluate and quantify specific proteins associated with disease risk. These scores are derived from the comprehensive analysis of protein expression patterns, post-translational modifications, and interactions within biological systems. By examining the proteome—the entire set of proteins in a cell, tissue, or organism—researchers aim to identify unique signatures or profiles that correlate with the likelihood of developing certain medical conditions, such as cancer or cardiovascular diseases. The integration of proteomic data enables the creation of predictive models, assigning individuals personalized risk scores based on their protein profiles. This innovative methodology holds significant promise for enhancing early disease detection, prognosis, and the development of targeted therapeutic interventions, ultimately contributing to more effective and personalized healthcare strategies.
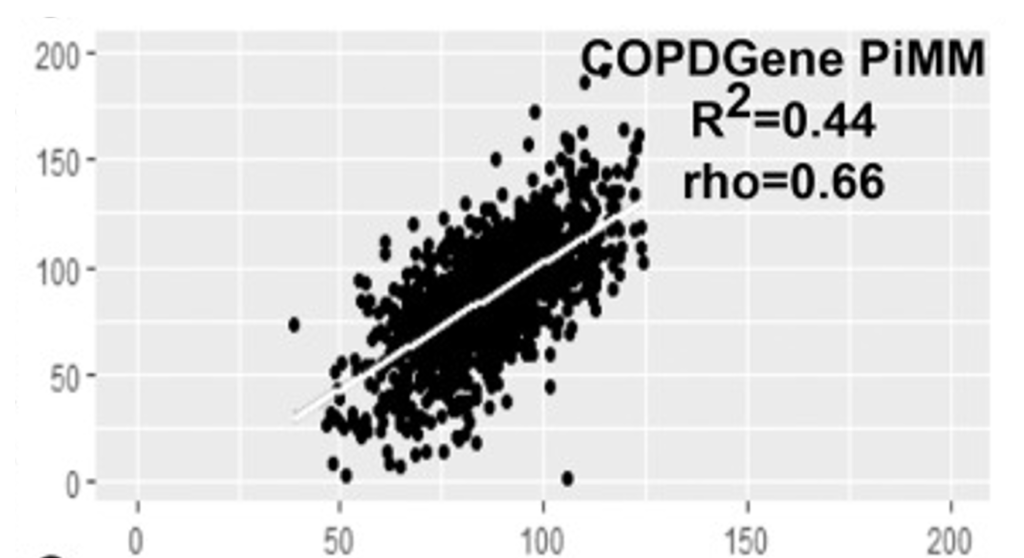
Key references from COPDGene research:
1. Bowler, R.P., T.M. Bahr, G. Hughes, S. Lutz, Y.I. Kim, C.D. Coldren, N. Reisdorph, and K.J. Kechris, Integrative omics approach identifies interleukin-16 as a biomarker of emphysema. OMICS, 2013. 17(12): p. 619-26.
2. Carolan, B.J., G. Hughes, J. Morrow, C.P. Hersh, W.K. O’Neal, S. Rennard, S.G. Pillai, P. Belloni, D.A. Cockayne, A.P. Comellas, M. Han, R.L. Zemans, K. Kechris, and R.P. Bowler, The association of plasma biomarkers with computed tomography-assessed emphysema phenotypes. Respir Res, 2014. 15(1): p. 127.
3. Dijkstra, A.E., J. Smolonska, M. van den Berge, C. Wijmenga, P. Zanen, M.A. Luinge, M. Platteel, J.W. Lammers, M. Dahlback, K. Tosh, P.S. Hiemstra, P.J. Sterk, A. Spira, J. Vestbo, B.G. Nordestgaard, M. Benn, S.F. Nielsen, M. Dahl, W.M. Verschuren, H.S. Picavet, H.A. Smit, M. Owsijewitsch, H.U. Kauczor, H.J. de Koning, E. Nizankowska-Mogilnicka, F. Mejza, P. Nastalek, C.C. van Diemen, M.H. Cho, E.K. Silverman, J.D. Crapo, T.H. Beaty, D.A. Lomas, P. Bakke, A. Gulsvik, Y. Bosse, M. Obeidat, D.W. Loth, L. Lahousse, F. Rivadeneira, A.G. Uitterlinden, A. Hofman, B.H. Stricker, G.G. Brusselle, C.M. van Duijn, U. Brouwer, G.H. Koppelman, J.M. Vonk, M.C. Nawijn, H.J. Groen, W. Timens, H.M. Boezen, D.S. Postma, and s. LifeLines Cohort, Susceptibility to chronic mucus hypersecretion, a genome wide association study. PLoS One, 2014. 9(4): p. e91621.
4. Roberts, M.E.P., B.W. Higgs, P. Brohawn, F. Pilataxi, X. Guo, M. Kuziora, R.P. Bowler, and W.I. White, CD4+ T-Cell Profiles and Peripheral Blood Ex-Vivo Responses to T-Cell Directed Stimulation Delineate COPD Phenotypes. Chronic Obstr Pulm Dis, 2015. 2(4): p. 268-280.
5. Sun, W., K. Kechris, S. Jacobson, M.B. Drummond, G.A. Hawkins, J. Yang, T.H. Chen, P.M. Quibrera, W. Anderson, R.G. Barr, P.V. Basta, E.R. Bleecker, T. Beaty, R. Casaburi, P. Castaldi, M.H. Cho, A. Comellas, J.D. Crapo, G. Criner, D. Demeo, S.A. Christenson, D.J. Couper, J.L. Curtis, C.M. Doerschuk, C.M. Freeman, N.A. Gouskova, M.K. Han, N.A. Hanania, N.N. Hansel, C.P. Hersh, E.A. Hoffman, R.J. Kaner, R.E. Kanner, E.C. Kleerup, S. Lutz, F.J. Martinez, D.A. Meyers, S.P. Peters, E.A. Regan, S.I. Rennard, M.B. Scholand, E.K. Silverman, P.G. Woodruff, W.K. O’Neal, R.P. Bowler, S.R. Group, and C.O. Investigators, Common Genetic Polymorphisms Influence Blood Biomarker Measurements in COPD. PLoS Genet, 2016. 12(8): p. e1006011.
6. Bradford, E., S. Jacobson, J. Varasteh, A.P. Comellas, P. Woodruff, W. O’Neal, D.L. DeMeo, X. Li, V. Kim, M. Cho, P.J. Castaldi, C. Hersh, E.K. Silverman, J.D. Crapo, K. Kechris, and R.P. Bowler, The value of blood cytokines and chemokines in assessing COPD. Respir Res, 2017. 18(1): p. 180.
7. Zemans, R.L., S. Jacobson, J. Keene, K. Kechris, B.E. Miller, R. Tal-Singer, and R.P. Bowler, Multiple biomarkers predict disease severity, progression and mortality in COPD. Respir Res, 2017. 18(1): p. 117.
8. Regan, E.A., C.P. Hersh, P.J. Castaldi, D.L. DeMeo, E.K. Silverman, J.D. Crapo, and R.P. Bowler, Omics and the Search for Blood Biomarkers in Chronic Obstructive Pulmonary Disease. Insights from COPDGene. Am J Respir Cell Mol Biol, 2019. 61(2): p. 143-149.
9. Young, K.A., E.A. Regan, M.K. Han, S.M. Lutz, M. Ragland, P.J. Castaldi, G.R. Washko, M.H. Cho, M. Strand, D. Curran-Everett, T.H. Beaty, R.P. Bowler, E.S. Wan, D.A. Lynch, B.J. Make, E.K. Silverman, J.D. Crapo, J.E. Hokanson, G.L. Kinney, and C.O. Investigators, Subtypes of COPD Have Unique Distributions and Differential Risk of Mortality. Chronic Obstr Pulm Dis, 2019. 6(5): p. 400-413.
10. Mastej, E., L. Gillenwater, Y. Zhuang, K.A. Pratte, R.P. Bowler, and K. Kechris, Identifying Protein-metabolite Networks Associated with COPD Phenotypes. Metabolites, 2020. 10(4).
11. Raffield, L.M., H. Dang, K.A. Pratte, S. Jacobson, L.A. Gillenwater, E. Ampleford, I. Barjaktarevic, P. Basta, C.B. Clish, A.P. Comellas, E. Cornell, J.L. Curtis, C. Doerschuk, P. Durda, C. Emson, C.M. Freeman, X. Guo, A.T. Hastie, G.A. Hawkins, J. Herrera, W.C. Johnson, W.W. Labaki, Y. Liu, B. Masters, M. Miller, V.E. Ortega, G. Papanicolaou, S. Peters, K.D. Taylor, S.S. Rich, J.I. Rotter, P. Auer, A.P. Reiner, R.P. Tracy, D. Ngo, R.E. Gerszten, W.K. O’Neal, R.P. Bowler, and N.T.-O.f.P.M. Consortium, Comparison of Proteomic Assessment Methods in Multiple Cohort Studies. Proteomics, 2020. 20(12): p. e1900278.
12. Carpenter, C.M., W. Zhang, L. Gillenwater, C. Severn, T. Ghosh, R. Bowler, K. Kechris, and D. Ghosh, PaIRKAT: A pathway integrated regression-based kernel association test with applications to metabolomics and COPD phenotypes. PLoS Comput Biol, 2021. 17(10): p. e1008986.
13. Gillenwater, L.A., S. Helmi, E. Stene, K.A. Pratte, Y. Zhuang, R.P. Schuyler, L. Lange, P.J. Castaldi, C.P. Hersh, F. Banaei-Kashani, R.P. Bowler, and K.J. Kechris, Multi-omics subtyping pipeline for chronic obstructive pulmonary disease. PLoS One, 2021. 16(8): p. e0255337.
14. Pratte, K.A., J.L. Curtis, K. Kechris, D. Couper, M.H. Cho, E.K. Silverman, D.L. DeMeo, F.C. Sciurba, Y. Zhang, V.E. Ortega, W.K. O’Neal, L.A. Gillenwater, D.A. Lynch, E.A. Hoffman, J.D. Newell, Jr., A.P. Comellas, P.J. Castaldi, B.E. Miller, S.D. Pouwels, N. Hacken, R. Bischoff, F. Klont, P.G. Woodruff, R. Paine, R.G. Barr, J. Hoidal, C.M. Doerschuk, J.P. Charbonnier, R. Sung, N. Locantore, J.G. Yonchuk, S. Jacobson, R. Tal-Singer, D. Merrill, and R.P. Bowler, Soluble receptor for advanced glycation end products (sRAGE) as a biomarker of COPD. Respir Res, 2021. 22(1): p. 127.
15. Axelsson, G.T., G. Gudmundsson, K.A. Pratte, T. Aspelund, R.K. Putman, J.L. Sanders, E.F. Gudmundsson, H. Hatabu, V. Gudmundsdottir, A. Gudjonsson, T. Hino, T. Hida, B.D. Hobbs, M.H. Cho, E.K. Silverman, R.P. Bowler, L.J. Launer, L.L. Jennings, G.M. Hunninghake, V. Emilsson, and V. Gudnason, The Proteomic Profile of Interstitial Lung Abnormalities. Am J Respir Crit Care Med, 2022. 206(3): p. 337-346.
16. Gregory, A., Z. Xu, K. Pratte, S. Lee, C. Liu, R. Chase, J. Yun, A. Saferali, C.P. Hersh, R. Bowler, E. Silverman, P.J. Castaldi, and A. Boueiz, Clustering-based COPD subtypes have distinct longitudinal outcomes and multi-omics biomarkers. BMJ Open Respir Res, 2022. 9(1).
17. Klont, F., P. Horvatovich, R.P. Bowler, E. van Rikxoort, J.P. Charbonnier, M. Kwiatkowski, D.A. Lynch, S. Humphries, R. Bischoff, N.H.T. Ten Hacken, and S.D. Pouwels, Plasma sRAGE levels strongly associate with centrilobular emphysema assessed by HRCT scans. Respir Res, 2022. 23(1): p. 15.
18. Palzer, E.F., C.H. Wendt, R.P. Bowler, C.P. Hersh, S.E. Safo, and E.F. Lock, sJIVE: Supervised Joint and Individual Variation Explained. Comput Stat Data Anal, 2022. 175.
19. Serban, K.A., K.A. Pratte, C. Strange, R.A. Sandhaus, A.M. Turner, T. Beiko, D.A. Spittle, L. Maier, N. Hamzeh, E.K. Silverman, B.D. Hobbs, C.P. Hersh, D.L. DeMeo, M.H. Cho, and R.P. Bowler, Unique and shared systemic biomarkers for emphysema in Alpha-1 Antitrypsin deficiency and chronic obstructive pulmonary disease. EBioMedicine, 2022. 84: p. 104262.
20. Hill, A.C., C. Guo, E.M. Litkowski, A.W. Manichaikul, B. Yu, I.R. Konigsberg, B.A. Gorbet, L.A. Lange, K.A. Pratte, K.J. Kechris, M. DeCamp, M. Coors, V.E. Ortega, S.S. Rich, J.I. Rotter, R.E. Gerzsten, C.B. Clish, J.L. Curtis, X. Hu, M.E. Obeidat, M. Morris, J. Loureiro, D. Ngo, W.K. O’Neal, D.A. Meyers, E.R. Bleecker, B.D. Hobbs, M.H. Cho, F. Banaei-Kashani, and R.P. Bowler, Large scale proteomic studies create novel privacy considerations. Sci Rep, 2023. 13(1): p. 9254.
21. Suryadevara, R., A. Gregory, R. Lu, Z. Xu, A. Masoomi, S.M. Lutz, S. Berman, J.H. Yun, A. Saferali, M.H. Ryu, M. Moll, D.D. Sin, C.P. Hersh, E.K. Silverman, J. Dy, K.A. Pratte, R.P. Bowler, P.J. Castaldi, A. Boueiz, and C.O. investigators, Blood-based Transcriptomic and Proteomic Biomarkers of Emphysema. Am J Respir Crit Care Med, 2023.
Metabolomics
What is metabolomics? Metabolomics research is the investigation into the small molecules or metabolites that make up our body’s chemical fingerprint. Think of these molecules as the building blocks and energy sources that keep our bodies running smoothly. Scientists use advanced techniques to analyze and understand the unique combination of metabolites in different tissues or fluids, helping them uncover important information about our health. It’s a bit like studying the specific ingredients in a recipe to understand how it affects the final dish. Metabolomics can reveal changes in our body’s chemistry linked to various conditions, such as diabetes or heart disease, offering valuable insights that may lead to new ways of diagnosing, treating, and preventing diseases by getting to the root of our body’s biochemical story.
COPDGene is helping us discover the small molecules that cause emphysema.
COPDGene has been instrumental in identifying some key metabolic markers that have been studied in relation to COPD:
- Energy Metabolism: Disturbances in glucose metabolism and insulin resistance have been observed in individuals with COPD. Elevated blood glucose levels may be associated with the severity of the disease. Carnitine is also a COPD biomarker, particularly in women.
- Lipid Metabolism: Altered lipid metabolism, including changes in levels of triglycerides and cholesterol, has been reported in COPD patients. Lipid abnormalities may contribute to systemic inflammation and the progression of the disease. Sphingolipids are one of the leading lipid biomarkers of COPD. These
- Amino Acid Metabolism: Changes in amino acid profiles have been identified in COPD. Amino acids are the building blocks of proteins, and their alterations may reflect underlying metabolic dysregulation.
- Oxidative Stress Markers: Metabolic markers associated with oxidative stress, such as changes in levels of antioxidants and reactive oxygen species, have been studied in COPD. Oxidative stress plays a role in the inflammatory processes seen in COPD. Ergothioneine is a naturally occurring amino acid derivative that acts as an antioxidant. It is unique in its structure and is not synthesized by the human body. Instead, it is obtained through the diet, primarily from certain foods. Ergothioneine is often found in relatively high concentrations in certain mushrooms, such as shiitake and oyster mushrooms, as well as some other foods like black beans and garlic. One of the notable features of ergothioneine is its ability to protect cells from oxidative stress. Oxidative stress occurs when there is an imbalance between the production of reactive oxygen species (free radicals) and the body’s ability to neutralize them with antioxidants. Ergothioneine is believed to play a role in mitigating this stress by scavenging free radicals and helping to maintain cellular health. While research on ergothioneine is ongoing, its potential health benefits and precise mechanisms of action are still being explored. It is an interesting compound in the field of nutrition and health due to its antioxidant properties and its presence in various dietary sources.
![]() |
Figure 2. Metabolite associations with COPD. (A) Heat map of signed p-values. Metabolites are organized by super pathway. Red intensity indicates positive direction of effect, while blue intensity indicates negative. Only select metabolites most significantly associated are labeled. (B) Scatter plot of 1-stearoyl-2-linoleoyl-GPI (18:0/18:2) abundance by FEV1/FVC ratio. (C) Scatter plot of phosphocholine abundance by FEV1pp. (D) Bee swarm of ergothioneine abundance by GOLD stage. Ergothioneine was one of the topmost associated metabolites with all airflow obstruction phenotypes. Metabolites are color coded by Super Pathway designation. Metabolite abundances are inverse normal transformed. *Indicates compounds that have not been officially confirmed based on a standard, but Metabolon is confident in its identity. COPD, chronic obstructive pulmonary disease; FEV1, forced expiratory volume at one second; FEV1pp, FEV1 percent predicted; FVC, forced vital capacity; GPI, glycophosphatidylinositol. |
There are differences between men and women for the metabolite signatures of COPD.
COPD is different between men and women. COPDGene helped discover some of those different risk factors. For instance, sphingolipids are typically higher in men with COPD compared to women but carnitines are typically higher in women with COPD compared to men (Figure 3).
![]() |
Figure 3. Scatter plot of sex specific betas for COPD models in COPDGene. The x-axis represents the beta estimates in the female stratum while the y-axis represents the beta estimates in the male stratum. Point shape corresponds with module assignment. Points are colored by significance in specific strata |
Key references in which COPDGene was instrumental in identifying metabolomic markers of COPD:
- Carpenter, C.M., L. Gillenwater, R. Bowler, K. Kechris, and D. Ghosh, TreeKernel: interpretable kernel machine tests for interactions between -omics and clinical predictors with applications to metabolomics and COPD phenotypes. BMC Bioinformatics, 2023. 24(1): p. 398.
- Godbole, S., W.W. Labaki, K.A. Pratte, A. Hill, M. Moll, A.T. Hastie, S.P. Peters, A. Gregory, V.E. Ortega, D. DeMeo, M.H. Cho, S.P. Bhatt, J.M. Wells, I. Barjaktarevic, K.A. Stringer, A. Comellas, W. O’Neal, K. Kechris, and R.P. Bowler, A Metabolomic Severity Score for Airflow Obstruction and Emphysema. Metabolites, 2022. 12(5).
- Gillenwater, L.A., K.J. Kechris, K.A. Pratte, N. Reisdorph, I. Petrache, W.W. Labaki, W. O’Neal, J.A. Krishnan, V.E. Ortega, D.L. DeMeo, and R.P. Bowler, Metabolomic Profiling Reveals Sex Specific Associations with Chronic Obstructive Pulmonary Disease and Emphysema. Metabolites, 2021. 11(3).
- Gillenwater, L.A., S. Helmi, E. Stene, K.A. Pratte, Y. Zhuang, R.P. Schuyler, L. Lange, P.J. Castaldi, C.P. Hersh, F. Banaei-Kashani, R.P. Bowler, and K.J. Kechris, Multi-omics subtyping pipeline for chronic obstructive pulmonary disease. PLoS One, 2021. 16(8): p. e0255337.
- Carpenter, C.M., W. Zhang, L. Gillenwater, C. Severn, T. Ghosh, R. Bowler, K. Kechris, and D. Ghosh, PaIRKAT: A pathway integrated regression-based kernel association test with applications to metabolomics and COPD phenotypes. PLoS Comput Biol, 2021. 17(10): p. e1008986.
- Raffield, L.M., H. Dang, K.A. Pratte, S. Jacobson, L.A. Gillenwater, E. Ampleford, I. Barjaktarevic, P. Basta, C.B. Clish, A.P. Comellas, E. Cornell, J.L. Curtis, C. Doerschuk, P. Durda, C. Emson, C.M. Freeman, X. Guo, A.T. Hastie, G.A. Hawkins, J. Herrera, W.C. Johnson, W.W. Labaki, Y. Liu, B. Masters, M. Miller, V.E. Ortega, G. Papanicolaou, S. Peters, K.D. Taylor, S.S. Rich, J.I. Rotter, P. Auer, A.P. Reiner, R.P. Tracy, D. Ngo, R.E. Gerszten, W.K. O’Neal, R.P. Bowler. Consortium, Comparison of Proteomic Assessment Methods in Multiple Cohort Studies. Proteomics, 2020. 20(12): p. e1900278.
- Mastej, E., L. Gillenwater, Y. Zhuang, K.A. Pratte, R.P. Bowler, and K. Kechris, Identifying Protein-metabolite Networks Associated with COPD Phenotypes. Metabolites, 2020. 10(4).
- Gillenwater, L.A., K.A. Pratte, B.D. Hobbs, M.H. Cho, Y. Zhuang, E. Halper-Stromberg, C. Cruickshank-Quinn, N. Reisdorph, I. Petrache, W.W. Labaki, W.K. O’Neal, V.E. Ortega, D.P. Jones, K. Uppal, S. Jacobson, G. Michelotti, C.H. Wendt, K.J. Kechris, and R.P. Bowler, Plasma Metabolomic Signatures of Chronic Obstructive Pulmonary Disease and the Impact of Genetic Variants on Phenotype-Driven Modules. Netw Syst Med, 2020. 3(1): p. 159-181.
- Regan, E.A., C.P. Hersh, P.J. Castaldi, D.L. DeMeo, E.K. Silverman, J.D. Crapo, and R.P. Bowler, Omics and the Search for Blood Biomarkers in Chronic Obstructive Pulmonary Disease. Insights from COPDGene. Am J Respir Cell Mol Biol, 2019. 61(2): p. 143-149.
- Bowler, R.P., S. Jacobson, C. Cruickshank, G.J. Hughes, C. Siska, D.S. Ory, I. Petrache, J.E. Schaffer, N. Reisdorph, and K. Kechris, Plasma sphingolipids associated with chronic obstructive pulmonary disease phenotypes. Am J Respir Crit Care Med, 2015. 191(3): p. 275-84.
- Bahr, T.M., G.J. Hughes, M. Armstrong, R. Reisdorph, C.D. Coldren, M.G. Edwards, C. Schnell, R. Kedl, D.J. LaFlamme, N. Reisdorph, K.J. Kechris, and R.P. Bowler, Peripheral blood mononuclear cell gene expression in chronic obstructive pulmonary disease. Am J Respir Cell Mol Biol, 2013. 49(2): p. 316-23.
Transcriptomics
What is transcriptomics: Genes affect our health through the process of gene expression, in which DNA tells our cells what proteins to make through the creation of messenger RNA (mRNAs). New technologies allow us to measure all of the genes that are being expressed as mRNAs in our blood or other tissues, giving a snapshot of the biological activity of our cells at a given point in time. The study of these gene expression measurements is called transcriptomics, and scientists in COPDGene have used transcriptomics to identify important biological processes that happen in COPD so that we can develop new treatments to reverse those harmful processes.
Identifying markers of increased risk of worsening lung function in COPD. COPDGene researchers have identified patterns in DNA and blood transcriptomics that can identify subjects with COPD and that may predict future risk of rapid loss of lung function in COPD. Because these transcriptomic signatures also indicate certain biological processes that cause COPD, further research is being performed to identify harmful patterns of inflammation in groups of people whose disease gets worse over time. We are hopeful that this approach will allow researchers to develop new treatments for COPD that can treat the right people at the right time to improve their lung health.
Identifying causes of COPD and COPD exacerbations. Some people with COPD experience bouts of cough and shortness of breath called COPD exacerbations, which can require antibiotics and hospitalization in severe cases. COPDGene researchers studying blood transcriptomics identified a pattern of inflammation called the interferon response that is higher in people who have more COPD exacerbations. The interferon response, which is important for fighting viral infections, is now understood to be a marker for higher risk for exacerbations and loss of lung function in the future. Future work will investigate if treatments targeting the interferon response may be beneficial for people with COPD. We can also use the gene expression information to estimate the numbers of immune cells in the blood. Using these methods, we found that people with lower numbers of a subset of T cells in the blood had a higher risk for COPD exacerbations.
Understanding the biological effects of smoking and vaping. Smoking is a major cause of COPD, heart disease, and many cancers. COPDGene researchers have used blood transcriptomics to identify how smoking affects our immune system, and they have discovered new links between smoking and a biological process called alternative splicing. Since some smokers are more likely to develop COPD than others, ongoing work in COPDGene is focused on identifying which smokers are at the highest risk of developing COPD and why that occurs. E-cigarettes, or vaping, is another exposure that has increased in frequency, and researchers in COPDGene have studied the biological effects of vaping and compared these to the effects of smoking. This work will help to understand the relative risks of smoking and vaping, and what role if any may be appropriate for vaping as a way to reduce traditional cigarette exposure.
Key references from COPDGene transcriptomics research:
Boueiz, Adel, Betty Pham, Robert Chase, Andrew Lamb, Sool Lee, Zun Zar Chi Naing, Michael H. Cho, et al. 2019. “Integrative Genomics Analysis Identifies ACVR1B as a Candidate Causal Gene of Emphysema Distribution.” American Journal of Respiratory Cell and Molecular Biology 60 (4): 388–98.
Buschur, Kristina L., Craig Riley, Aabida Saferali, Peter Castaldi, Grace Zhang, Francois Aguet, Kristin G. Ardlie, et al. 2023. “Distinct COPD Subtypes in Former Smokers Revealed by Gene Network Perturbation Analysis.” Respiratory Research 24 (1): 30.
Chen, Junxiang, Zhonghui Xu, Li Sun, Ke Yu, Craig P. Hersh, Adel Boueiz, John E. Hokanson, et al. 2023. “Deep Learning Integration of Chest Computed Tomography Imaging and Gene Expression Identifies Novel Aspects of COPD.” International Journal of Chronic Obstructive Pulmonary Disease, June. https://doi.org/10.15326/jcopdf.2023.0399.
Ghosh, Auyon J., Aabida Saferali, Sool Lee, Robert Chase, Matthew Moll, Jarrett Morrow, Jeong Yun, Peter J. Castaldi, and Craig P. Hersh. 2022. “Blood RNA Sequencing Shows Overlapping Gene Expression across COPD Phenotype Domains.” Thorax 77 (2): 115–22.
Gregory, Andrew, Zhonghui Xu, Katherine Pratte, Seth Berman, Robin Lu, Rahul Suryadevara, Robert Chase, et al. 2023. “Blood RNA and Protein Biomarkers Are Associated with Vaping and Dual Use, and Prospective Health Outcomes.” F1000Research 12 (123): 123.
Moll, Matthew, Adel Boueiz, Auyon J. Ghosh, Aabida Saferali, Sool Lee, Zhonghui Xu, Jeong H. Yun, et al. 2022. “Development of a Blood-Based Transcriptional Risk Score for Chronic Obstructive Pulmonary Disease.” American Journal of Respiratory and Critical Care Medicine 205 (2): 161–70.
Morrow, Jarrett D., Robert P. Chase, Margaret M. Parker, Kimberly Glass, Minseok Seo, Miguel Divo, Caroline A. Owen, et al. 2019. “RNA-Sequencing across Three Matched Tissues Reveals Shared and Tissue-Specific Gene Expression and Pathway Signatures of COPD.” Respiratory Research 20 (1): 65.
Parker, Margaret M., Robert P. Chase, Andrew Lamb, Alejandro Reyes, Aabida Saferali, Jeong H. Yun, Blanca E. Himes, Edwin K. Silverman, Craig P. Hersh, and Peter J. Castaldi. 2017. “RNA Sequencing Identifies Novel Non-Coding RNA and Exon-Specific Effects Associated with Cigarette Smoking.” BMC Medical Genomics 10 (1): 58.
Ryu, Min Hyung, Jeong H. Yun, Jarrett D. Morrow, Aabida Saferali, Peter Castaldi, Robert Chase, Meryl Stav, et al. 2023. “Blood Gene Expression and Immune Cell Subtypes Associated with Chronic Obstructive Pulmonary Disease Exacerbations.” American Journal of Respiratory and Critical Care Medicine 208 (3): 247–55.
Saferali, Aabida, Jeong H. Yun, Margaret M. Parker, Phuwanat Sakornsakolpat, Robert P. Chase, Andrew Lamb, Brian D. Hobbs, et al. 2019. “Analysis of Genetically Driven Alternative Splicing Identifies FBXO38 as a Novel COPD Susceptibility Gene.” PLoS Genetics 15 (7): e1008229.
Wang, Zifeng, Aria Masoomi, Zhonghui Xu, Adel Boueiz, Sool Lee, Tingting Zhao, Russell Bowler, et al. 2021. “Improved Prediction of Smoking Status via Isoform-Aware RNA-Seq Deep Learning Models.” PLoS Computational Biology 17 (10): e1009433.
Xu, Zhonghui, John Platig, Sool Lee, Adel Boueiz, Rob Chase, Dhawal Jain, Andrew Gregory, et al. 2021. “Cigarette Smoking-Associated Isoform Switching and 3’ UTR Lengthening via Alternative Polyadenylation.” Genomics 113 (6): 4184–95.
Yun, Jeong H., Robert Chase, Margaret M. Parker, Aabida Saferali, Peter J. Castaldi, Edwin K. Silverman, and Craig P. Hersh. 2019. “Peripheral Blood Gene Expression Signatures of Eosinophilic Chronic Obstructive Pulmonary Disease.” American Journal of Respiratory Cell and Molecular Biology 61 (3): 398–401.
Yun, Jeong H., Sool Lee, Pooja Srinivasa, Jarrett Morrow, Robert Chase, Aadbida Saferali, Zhonghui Xu, Michael Cho, Peter Castaldi, and Craig P. Hersh. 2022. “An Interferon-Inducible Signature of Airway Disease from Blood Gene Expression Profiling.” The European Respiratory Journal: Official Journal of the European Society for Clinical Respiratory Physiology 59 (5). https://doi.org/10.1183/13993003.00569-2021.